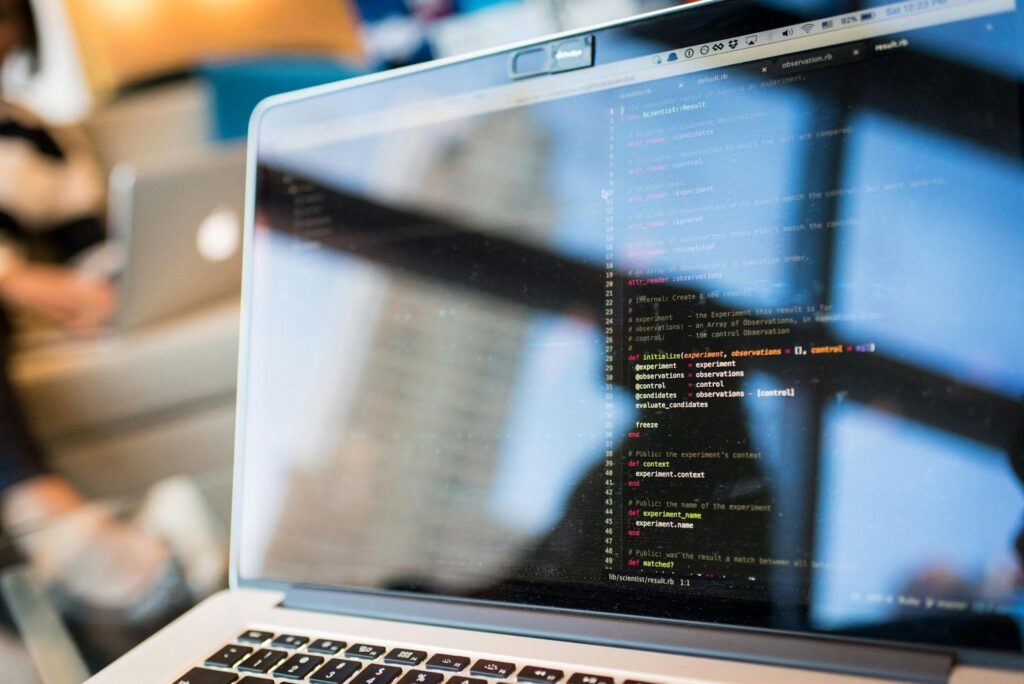
Imagine waking up to a home that has already brewed your coffee, adjusted the thermostat, and even scheduled your day based on the weather forecast. This is the promise of IoT (Internet of Things).
The Internet of Things (IoT) is rapidly transforming the landscape of both personal and business environments. From smart homes equipped with interconnected devices to complex industrial systems, IoT technology offers unprecedented convenience and efficiency. The convenience and efficiency of IoT are undeniable, but what happens behind the scenes to manage the vast amounts of data generated by these devices?
This article explores the challenges of data management in the IoT ecosystem and offers practical solutions.
Post Contents
The Explosion of IoT Devices
The growth of IoT devices has been nothing short of phenomenal. With billions of devices connected worldwide, the data they generate is staggering. Each smart device, from your fitness tracker to industrial sensors, constantly collects and transmits data. This data overload presents a significant challenge. How do we handle such a massive influx of information efficiently?
Data overload is a real issue. Traditional data management systems struggle to cope with the sheer volume, variety, and velocity of IoT data. The result is often data silos, where information is stored in isolated pockets, making it difficult to access and analyze comprehensively.
Addressing IoT Challenges
Master data management provides a structured approach to addressing the challenges associated with IoT data. MDM involves creating a single, consistent, and accurate source of truth for critical data across an organization. In the context of IoT, MDM ensures that data from various devices is standardized and integrated effectively.
Governance frameworks play a vital role in maintaining data quality, consistency, and security. These frameworks set the rules for how data is collected, stored, and accessed. However, implementing these frameworks can be challenging. Data silos, inconsistent data formats, and the need for real-time processing are common obstacles.
To overcome these challenges, organizations must adopt MDM strategies. Automated tools can help streamline data integration and management processes. Additionally, comprehensive data governance policies are essential. These policies should define data standards, establish data stewardship roles, and ensure compliance with regulations.
Data Security and Privacy Concerns
Security risks are a significant concern in the IoT landscape. With so many devices connected to the internet, each one is a potential entry point for cyber-attacks. Data breaches can result in the loss of sensitive information and damage to a company’s reputation.
Privacy issues are equally important. IoT devices often collect personal data, raising concerns about how this data is used and protected. Users need to trust that their information is secure and their privacy is respected.
Addressing these concerns requires a multifaceted approach. Best practices for securing IoT devices include encryption, regular software updates, and user education. Encryption ensures that data is protected during transmission and storage. Regular updates help fix vulnerabilities that cybercriminals might exploit. Educating users about security best practices can prevent common pitfalls, such as using weak passwords.
Interoperability and Standardization
Interoperability is another significant challenge. Different IoT devices and platforms often struggle to communicate with each other due to a lack of standardization. This fragmentation can hinder the seamless integration of IoT systems.
Promoting industry standards is one solution to this problem. Standards ensure that devices from different manufacturers can work together. Middleware solutions can also help by acting as a bridge between incompatible systems. When designing IoT devices, ensuring compatibility with existing standards can prevent interoperability issues down the line.
Data Storage and Processing Challenges
Storing and processing the vast amounts of data generated by IoT devices is no small feat. Traditional storage solutions may not be sufficient to handle the scale and speed required.
Modern storage solutions, such as distributed databases and cloud storage, offer better scalability and flexibility. These solutions can accommodate the growing volume of data without sacrificing performance. However, they come with their own set of challenges, such as latency and security concerns.
Edge computing and cloud computing offer different data processing benefits. Edge computing involves processing data closer to where it is generated, reducing latency and bandwidth usage. Cloud computing provides powerful processing capabilities and scalable storage. Combining these approaches can offer the best of both worlds. Data can be processed quickly at the edge, with more complex tasks handled in the cloud.
Using data compression techniques can also help manage storage needs. By reducing the size of data, organizations can store more information without requiring additional resources.
Data Analytics and Insights
The value of IoT data lies in the insights it can provide. Analyzing this data can help businesses make better decisions, improve efficiency, and create new opportunities. However, extracting meaningful insights from vast amounts of data is a challenge.
Real-time data processing is often necessary for IoT applications. For example, in smart cities, data from sensors must be analyzed immediately to manage traffic flow or respond to emergencies. Advanced analytics tools, including AI and machine learning algorithms, can help process data in real time and identify patterns and trends.
Implementing these tools requires skilled personnel who can interpret the data and extract actionable insights. Training and retaining such talent is crucial for organizations looking to leverage IoT data effectively.
Conclusion
The challenges of data management in the IoT ecosystem are significant, but they are not insurmountable. By understanding the issues and implementing practical solutions, organizations can harness the full potential of IoT.
Master Data Management and governance frameworks are essential for ensuring data quality and consistency. Security and privacy must be prioritized to protect sensitive information. Promoting interoperability and standardization can help integrate diverse IoT systems seamlessly. Modern storage solutions, edge computing, and cloud computing offer robust options for managing data storage and processing needs. Finally, advanced analytics tools and skilled personnel are key to unlocking valuable insights from IoT data.
As IoT continues to evolve, so too will the strategies for managing its data. Staying ahead of these challenges will be crucial for organizations looking to thrive in an increasingly connected world.